August 11, 2021
A Deep Dive into Deep Fakes
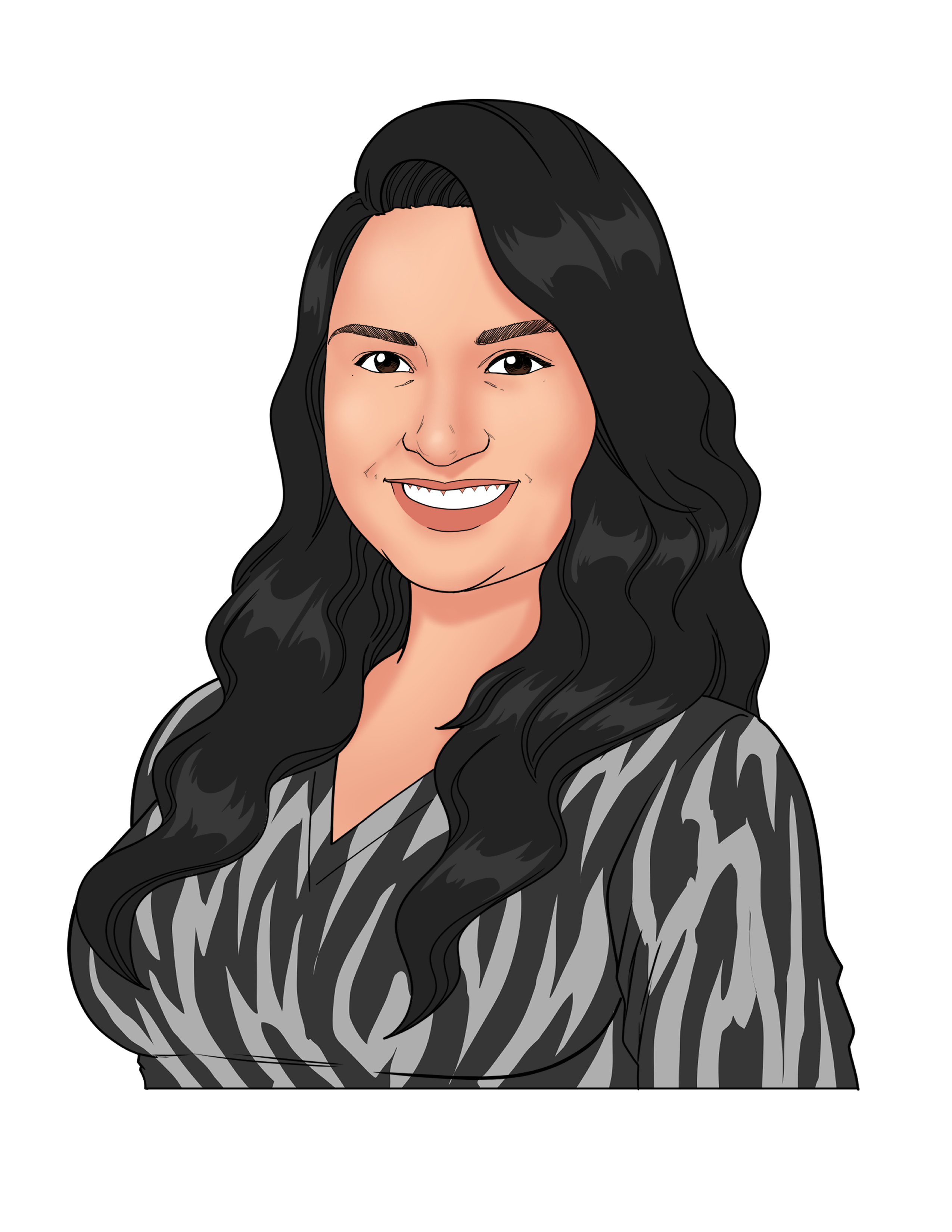
Malvika Mehta
Deep Fakes
Artificial intelligence (AI) and machine learning promise to evolve humanity to greater futuristic heights. The most pressing developments are in the areas of automation; AI-generated synthetic media, including audio or video, can bring unprecedented opportunities in the areas of art, education, and entertainment at a fraction of the cost. Deep fakes can replicate videos, change voices, and swap faces. The lip movements are accurate to the words spoken. Automated technology has introduced newer dimensions to overcome language barriers.
Films can be translated with original actors resulting in exponentially lower costs and labor-intensive manual processes. A quick render with the deep fake application can digitally alter humans like Samsung’s AI lab brought Mona Lisa’s smile to reality. The lab also created realistic videos of Marilyn Monroe, Albert Einstein, and Salvador Dali as if they were talking. Several deep fake examples can be found on the TikTok account dedicated to Tom Cruise @deeptomcruise. However, the technology escorts major threats potentially related to cyber warfare where poorly designed tools impose risks to hacking and provoke criminal use.
A Trojan Horse?
A survey conducted by Deep Trace Labs in June 2020 mentioned, the number of deep fake videos on the internet doubled within a period of just six months. They also reported that in 2019, 96% of the total deep fake videos consisted of non-consensual deep fake pornography. The target was almost exclusively women. The first deep fake video surfaced in 2017 where the face of a celebrity was swapped with the face of a porn actor. In such instances a detrimental rise in bullying scams, revenge porn can be perceived. Deep fake impersonations have started to have financial repercussions in society. The CEO of a UK-based energy firm fell prey to an approximately $243,000 USD scam. An AI-generated voice deep fake was used to impersonate the voice of the CEO’s boss.
Digital manipulations often lead to questioning the integrity of graphic media and the spread of fake news. Abuse of image manipulation techniques with easily available tools like Photoshop has led to the creation of photographs that misrepresent facts or media tailored to fulfill a person’s amusement. A picture is worth a thousand words, and in a world becoming increasingly more visual in pursuit of instant gratification, fake images could communicate more effectively than testimony in the eyes of a jury. Metadata can be manipulated or scrubbed, camera filters used on social media pages, and built-in applications can modify images to enhance facial features.
Deep fake videos are created by digitally altering a person's face with another individual. They can also be computer-generated. They are created with malign intentions to resemble a second person. It is one of the biggest threats posed by machine learning technology. The consequences include crafting sexually explicit content resembling political leaders, making film stars of common people. The content that floats on the internet is often taken at its face value, leaving damaged reputations in its wake. Words can be simply put into politician’s mouths to say things they never said, thereby altering elections as well as affecting national security.
How Are Deep Fakes Created?
Deep fakes need GANs (Generative Aversive Networks) and autoencoders, data is generated from scratch with graphical data, like images and music. GANs are used to create anime, face emojis, and even TikTok videos. Social media filters can change hairstyles, eye color, facial hair and even alter the age of the subject.
Matt Groh, a research assistant at the MIT Media Lab mentions the usage of facial recognition algorithms along with a deep learning computer network called variational auto-encoder (VAE). The creator would train a neural network of real footage or photographs of a person to gain a realistic understanding of their facial characteristics. How do they look from different angles? What are the lighting conditions?
Facial recognition algorithm captures different poses and natural lighting to the video frames. Deep learning trains the VAE to encode images that need to be swapped and decode the ones to be swapped with. It could be a computer-generated image (GAN) or belonging to another real human. GAN-generated graphics would then be superimposed to the real media by combining the encoder with the decoder.
A single photo of you found floating on the web is all it takes. Thispersondoesnotexist.com presents realistic images that are artificial (figure 1). The images are created using GAN and contain headshots from different ethnicities and ages. A website and an application called My Heritage uses AI to create moving videos from photographs (figure 2). It functions on the concept of Deep Nostalgia which animates still images and creates high-quality videos. It has been used to bring the dead people back to life, digitally.
Figure 1: None of these pictures belong to real individuals. (Thispersondoesnotexist.com)
|
|
|
|
Figure 2: Animated video from still images using My Heritage app.
Further, AI-integrated platforms like ReFace, allow users to transpose faces into videos and GIFs relying on the GAN technology. For demonstration, two images were taken from one of the greatest TV shows of all time, Friends. The images were uploaded to the ReFace app. A random video with embedded audio was chosen from the samples to create deep fake videos.
|
|
Figure 3: Open-source images for creating deep fake videos for demonstration purposes.
Figure 3 demonstrates the conversion of still images into deep fake videos with audio recordings.
Other tools that are used to create deep fakes include:
- Zao
- Deepfakes web
- Deep Face Lab
- Face App
A software called ‘Deep Nudes’ removes clothes from photographs (restricted to women). It has been used widely to undress women by swapping private parts in less than twenty seconds. This is horrifying. Moreover, it has been used to maliciously spread non-consensual and coercive porn on the internet. The tool was tested with multiple images and the results were close to reality with the ones that showed more skin and had less clothes (figure 4).
Figure 4: How Deep nudes are created using still images with software like 'Deep Nude'. Disclaimer: the image is of a mannequin and for educational purposes only.
Poor results were fetched for the ones that showed little skin. The free version of the tool adds a watermark in several locations. However, the watermark from a paid subscription can easily be cropped or removed.
There have been several cybercrime cases where fictitious online profiles develop fraudulent romantic relationships with vulnerable women (known as catfishing) to extort money. Defamation cases have also been reported where women were harassed with such claims. It is becoming easier for people to create videos of people saying things they never said or did.
Investigating Deep Fakes & Deep Nudes
Deep fakes can be convincing to the naked eye. Upon careful observation, in the videos above, one can spot the fabricated videos by analyzing inconsistencies. Some of the tips and tricks to spot professionally designed deep fakes are as follows:
- Does the content in the video make sense? What is the intention and the message that it is giving? Investigators can often find motives behind such videos that raise red flags.
- Observe every facial feature: start with a broader approach while looking at the entire face. In such videos, facial transformation is always present. The face may appear smoother, fine lines and wrinkles may be reduced. Characteristics like eyes, chin, and lips may stretch, distort or unusually loosen up.
- Next, observe the eyes. Identify natural shadows and light in the video. Are the movements of the eyes and eyebrows in sync with the mouth? Is the size of the lips unusual?
- What about blinking? Does the skin appear too smooth or filtered?
- Next, pay attention to accessories, like glasses, and facial hair. Creating deep fake videos may be challenging with images that contain glasses because of glare. Facial hair manipulation is challenging and often results in images feeling off or strange. The phrase “go with your gut” applies here.
- Now, monitor the body language. Is the neck in synchronization with the facial expressions? Are the person’s gestures coordinating with their mouth movements? Does the body movement seem robotic and artificial?
- While investigating deep nudes, consider the lighting, the background, position of the nipples, angles, distortions, and underlying shadows.
An MIT research project has developed a platform called ‘Detect Fakes’ https://detectfakes.media.mit.edu/ which contains a combination of fabricated and genuine media (videos with audio, silent videos, just audio, etc.). It is a good platform to test and practice investigation skills related to Deep fakes!
OSINT Tools to Detect Deep Fakes
Investigating procedures would commonly involve investigation of:
- Source of the video: social media analysis and digital forensics play an important role. Tools focused on revealing or restoring EXIF data are particularly useful.
- Reverse image search tools, such as Google Image Search, Yandex, and TinEye Reverse Image Search may provide some insights on the image frames.
- Audio analysis tools like Audacity or Deep Fake Audio Detection tools https://github.com/dessa-oss/fake-voice-detection may be leveraged.
Sensity.ai
Sensity allows users to analyze files to detect threats concerning the manipulation of images or videos. Figure 5 is an example of how the tool detects manipulations from the demonstration videos.
|
|
Figure 5: Detection of deep fake videos using Sensity.ai.
However, the tool cannot detect facial swaps if the images have been modified strategically. It includes a process of removing the background from the image, swapping a royalty-free stock image with a GAN image, and even interchanging EXIF data can reduce the chances of its detection (figure 6).
|
Figure 6: Undetected face swap using Sensity.ai.
Deepfake-o-meter
Researchers at the University of Buffalo have created a deep fake spotting tool with about 94% success rate. It works on the physics of light reflections in the eyes. The tool examines mismatched reflective differences in the eyes. The reflections should have the same shape and size in both eyes.
Deepware.ai
Similar to Sensity.ai, Deepware is a Deep Fake scanner. However, the results are not satisfactory. Figure 7 exhibits the scan results, the tool has managed to spot 1 fake video out of 2, whereas, Sensity.ai could spot both. One interesting feature of Deepware is that it flags threat areas (“pred”).
|
|
Figure 7: Deep fake scanning with Deepware.ai.
Bridging the Gap with OSINT
Technological developments have created close to realistic images, audio, and videos detrimental to the privacy and security of individuals in a democratic world. While there are several benefits of AI technology and GAN in areas of marketing, clothing, theatre, and cinema, the atrocities of cybercrime spare none. Reputational sabotage runs parallel to the creation and distribution of deep fakes, especially for people whose lives revolve in the public sphere, such as political figures, celebrities, and athletes. In the future, it may very well be your friends, your neighbors, your co-workers, or even your family. Thus, deep fakes are not less than a trojan horse that disguises itself as legitimate.
When it comes to the identification of the creator of deep fakes, they may remain anonymous or even be part of state-funded campaigns. There is a visible gap related to the possibilities of deep fake detecting tools, evident from the examples above. There could be a likelihood of integrating such scanners on social media platforms that can automatically detect fake videos, fake images, and fake news. Tech giants including Microsoft, Google, Facebook, and Twitter are trying to pace up in order to stay ahead of the abuse of deep fakes. Facebook has partnered with Microsoft and launched a deep fake detection challenge. Facebook created deep fakes and encouraged participants to develop Open Source tools for detection, results can be found at.
Google launched an advanced program on detection of fake audio using its own Automatic Speaker Verification Spoof Challenge in 2019. Researchers were asked to submit countermeasures against fake audios. Fabula.ai owned by Twitter helps to spot fake news. A private company called ZeroFox introduced Deepstar (open-source contribution) that incorporates a plug-in that automates the acquisition of the video from a website and obtains frames from it. It is useful to train and compare results using deep learning technology. OSINT tools must be developed to work on synchronization techniques to detect movements of facial characteristics with that of audio.
Investigators must be trained to monitor and identify deep fakes. Educational concepts, such as MIT’s ‘Detect Fakes’, are encouraged. The threatening reality of what we can see today is just the tip of an iceberg. If left unchecked, it may threaten us all.